In the digital age, data has become the fuel that drives businesses and innovation forward. With the constant influx of data from various sources, the roles of data analysts and scientists have become crucial in making sense of this wealth of information. These professionals are responsible for unlocking insights, driving informed decisions, and shaping the future of diverse industries.
Data analysts and scientists are often viewed as interchangeable roles, but they actually have distinct yet interconnected responsibilities. In this article, we will delve into the world of data analysis and science, providing a comprehensive understanding of these professions. We will explore the vital functions they perform, the skills they require, and the impact they have on various sectors such as business, technology, research, and healthcare.
Role of a Data Analyst
Data analysts are like detectives in the digital world, meticulously sifting through mountains of information to unveil patterns, trends, and hidden narratives. Their main responsibility is to collect, clean, and analyze data to reveal valuable insights that can inform decision-making processes. Let’s take a closer look at the key roles and responsibilities of a data analyst.
Data Collection and Preparation
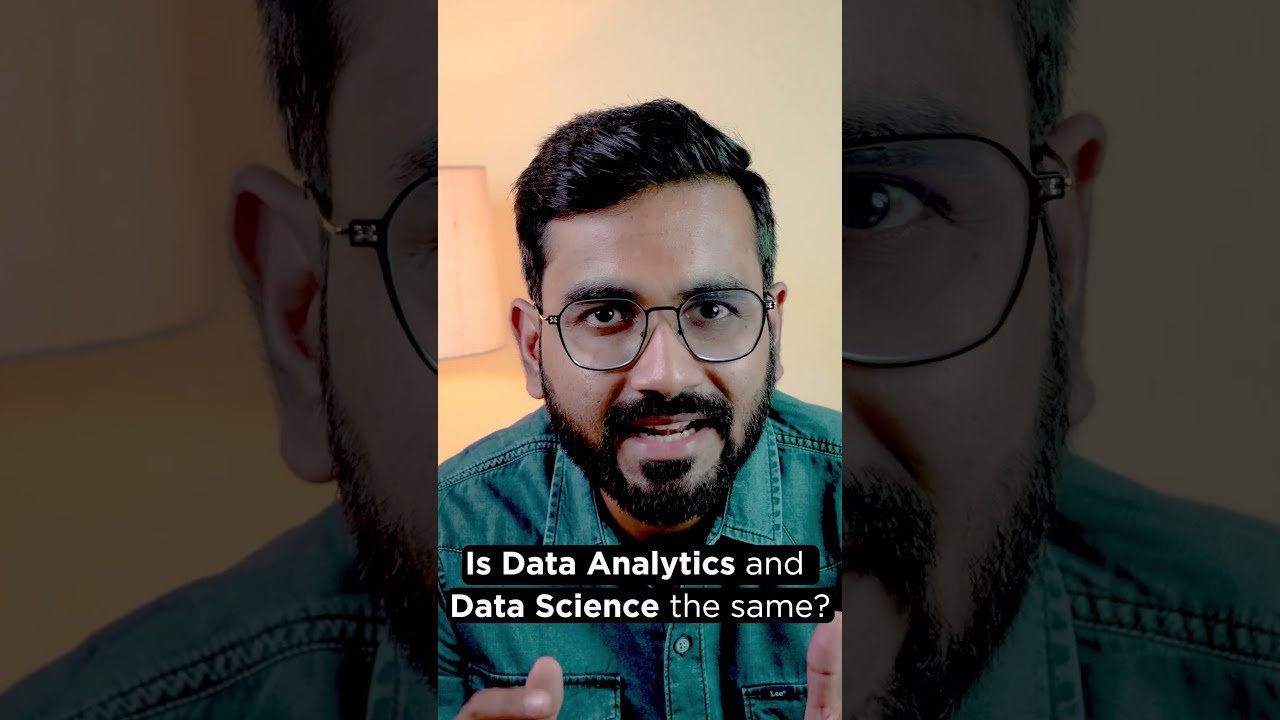
The first step in any data analysis project is data collection. Data analysts are skilled at gathering data from different sources, such as databases, spreadsheets, and online platforms. They also ensure that the data is accurate, complete, and consistent by performing data quality checks and resolving any discrepancies.
Once the data is collected, it needs to be prepared for analysis. This process involves cleaning, transforming, and structuring the data to make it usable for further analysis. Data analysts use tools and techniques such as data wrangling, data cleansing, and data formatting to ensure that the data is in a suitable format for analysis.
Data Analysis and Visualization

Once the data is collected and prepared, data analysts use various statistical methods and tools to analyze it. They apply techniques such as regression, clustering, and data mining to uncover patterns, trends, and correlations within the data. They also use advanced data visualization tools to present their findings in a visually appealing and easy-to-understand format.
Data analysts are also responsible for creating dashboards and reports that provide a summary of their analysis. These reports are crucial in conveying insights to stakeholders and informing decision-making processes within an organization.
Data Interpretation and Communication
One of the essential roles of a data analyst is to interpret the findings from their analysis and communicate them to non-technical audiences. This involves translating complex data into actionable insights and presenting them in a way that is understandable to business leaders and stakeholders. Effective communication skills are crucial for data analysts to bridge the gap between technical and non-technical teams.
Role of a Data Scientist
Data scientists have a broader scope of responsibilities compared to data analysts. They are responsible for both collecting and analyzing data as well as developing predictive models and algorithms. Data scientists are often involved in more complex and long-term projects that require advanced analytical skills. Let’s explore the key roles and responsibilities of a data scientist.
Data Collection and Preparation
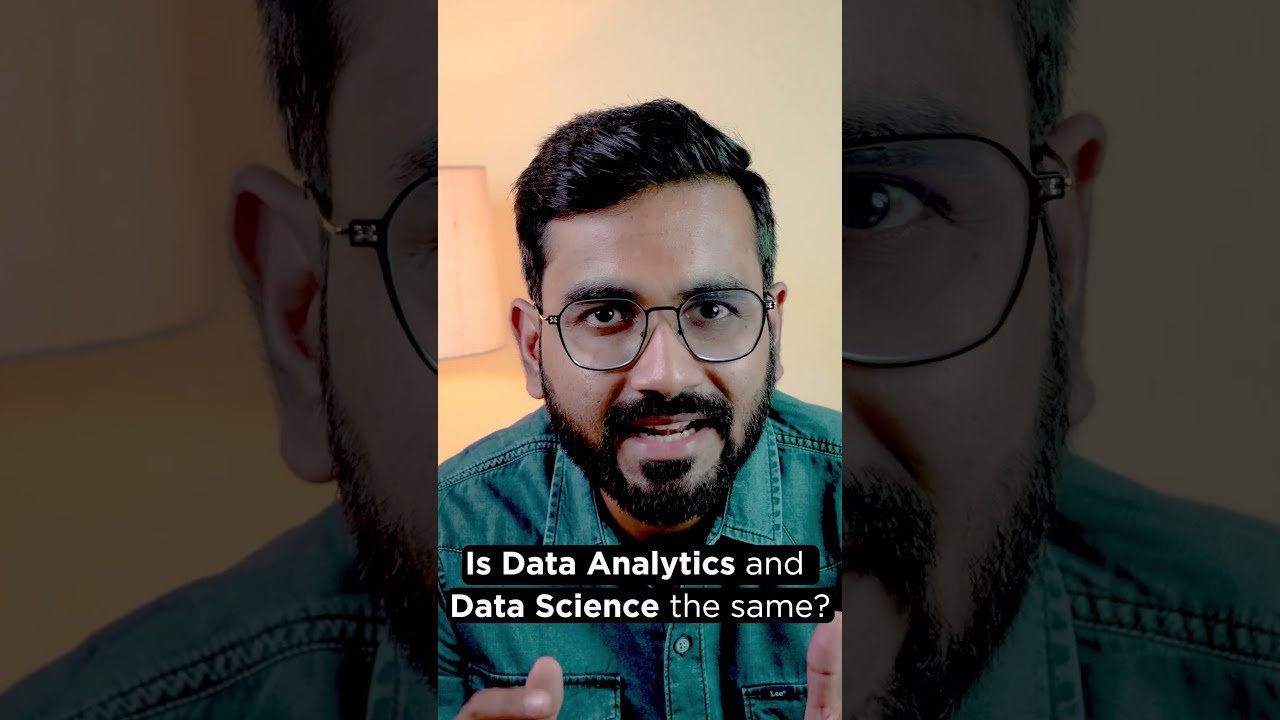
Similar to data analysts, data scientists are responsible for collecting and preparing data for analysis. However, they may also be involved in designing data collection methods and developing systems for data storage and retrieval.
Data scientists are skilled at using programming languages such as Python and R to extract and manipulate data from various sources. They also use tools like Hadoop and SQL to manage large datasets and ensure data quality.
Machine Learning and Predictive Modeling
Machine learning and predictive modeling are at the core of a data scientist’s role. These techniques involve using algorithms to analyze large datasets and make predictions or identify patterns. Data scientists apply these techniques to solve complex problems and develop data-driven solutions for businesses.
They also use machine learning algorithms to build predictive models that can be integrated into systems to automate decision-making processes. These models can help organizations make accurate and timely predictions based on past data.
Data Storytelling and Communication
Data scientists not only analyze and interpret data but also create compelling visualizations and infographics to tell a story from the data. They are skilled at using data storytelling techniques to communicate complex findings in a simple and engaging manner.
Data scientists also collaborate with data analysts and other team members to present the results of their analysis and provide recommendations for future actions.
Differences between Data Analyst and Data Scientist
While data analysts and scientists have some overlapping responsibilities, there are distinct differences between these roles. Here are some key differences between data analysts and data scientists:
Data Analyst | Data Scientist |
---|---|
Collects, cleans, and analyzes data | Involved in designing and developing data collection methods and systems |
Applies statistical methods and data visualization to uncover insights | Uses machine learning and predictive modeling to solve complex problems |
Communicates insights to non-technical audiences | Collaborates with cross-functional teams and communicates findings to technical and non-technical stakeholders |
Focuses on short-term projects | Involved in long-term projects that require advanced analytical skills |
Common Skills Required for Both Roles
Both data analysts and scientists require a mix of technical and soft skills to excel in their roles. While their specific skill sets differ, there are some common skills that both professionals should possess. Let’s take a look at some of these essential skills.
Technical Skills
- Proficiency in programming languages such as Python, R, SQL, and Java
- Knowledge of statistical methods and tools like regression, clustering, and data mining
- Familiarity with data visualization tools such as Tableau and Power BI
- Experience with big data technologies like Hadoop and Spark
- Understanding of databases, data warehousing, and data management systems
Analytical Skills
- Ability to identify patterns, trends, and correlations within data
- Critical thinking and problem-solving skills to tackle complex issues
- Attention to detail and the ability to spot anomalies and outliers in data
- Understanding of machine learning algorithms and predictive modeling techniques
- Ability to draw insights and make sound decisions based on data analysis
Soft Skills
- Excellent communication and presentation skills
- Teamwork and collaboration with cross-functional teams
- Time management and organization skills to meet project deadlines
- Adaptability and willingness to learn new tools and technologies
- Business acumen and understanding of the industry for which they are analyzing data.
How Data Analysts and Data Scientists Work Together
Data analysts and data scientists often work together on projects, leveraging each other’s skills and expertise to achieve a common goal. While their responsibilities may differ, the synergy between these roles is crucial in driving insights and making informed decisions. Here are some ways in which data analysts and data scientists collaborate:
Complementary Skill Sets
Data analysts and data scientists have different skill sets, but when combined, they can provide a comprehensive understanding of data. Data analysts bring their expertise in data collection, cleaning, and analysis, while data scientists use advanced analytical techniques to uncover patterns and make predictions. Together, they can provide valuable insights that inform business strategy and decision-making processes.
Interdisciplinary Teams
In today’s fast-paced and data-driven world, it’s becoming increasingly common for organizations to have interdisciplinary teams that include both data analysts and data scientists. These teams are comprised of professionals from different backgrounds, including business, technology, and data science, who work together to analyze data and drive innovation.
Cross-Functional Collaboration
Data analysts and data scientists also collaborate with other departments within an organization to gain a holistic understanding of the data. This may include working with marketing teams to understand customer behavior or collaborating with product teams to identify opportunities for improvement. By working together with various departments, data analysts and data scientists can provide valuable insights that drive the organization forward.
Importance of Collaboration between Data Analyst and Data Scientist
The collaboration between data analysts and data scientists is crucial for the success of data-driven projects. By combining their skills and expertise, they can provide a more comprehensive understanding of data, leading to better decision-making and improved business outcomes. Here are some reasons why collaboration between these two roles is essential:
Holistic Understanding of Data
Data analysts and data scientists have different areas of expertise, but when combined, they can provide a more holistic understanding of data. While data analysts focus on collecting and analyzing data, data scientists bring their predictive modeling skills to the table. Together, they can provide a complete picture of the data, uncovering insights that may not be apparent to just one role alone.
A More Comprehensive Approach
Collaboration between data analysts and data scientists allows for a more comprehensive approach to tackling complex problems. Data analysts may identify patterns and trends in the data, but data scientists can use their machine learning skills to build predictive models that can provide valuable insights for future strategies. By working together, these professionals can leverage each other’s skills to provide a more in-depth analysis of the data.
Faster and More Accurate Results
By collaborating, data analysts and data scientists can divide the workload and work on different aspects of a project simultaneously. This leads to faster results, allowing organizations to make timely decisions based on accurate data. Moreover, by working together, they can also double-check each other’s work, reducing the chances of error and ensuring the accuracy of results.
Case Studies
To further understand the impact of data analysts and data scientists, let’s take a look at a couple of case studies from different industries.
Retail Industry – Walmart
Walmart, one of the world’s largest retailers, uses data analysis and prediction algorithms to improve its inventory management. They collect data from various sources such as sales, weather forecasts, and social media, and use predictive modeling techniques to anticipate demand for certain products. This allows them to optimize their inventory levels, reduce costs, and improve customer satisfaction.
Additionally, Walmart also uses data analysis to identify buying patterns and trends among their customers. This information is then used to personalize offers and promotions, resulting in increased sales and customer loyalty.
Healthcare Industry – Cerner Corporation
Cerner Corporation is a leading provider of electronic health record systems and other healthcare technology solutions. They use data analysis and predictive modeling to help healthcare providers make informed decisions about patient care and population health.
Their HealtheIntent platform collects and analyzes data from various sources such as electronic health records, clinical data, and consumer data. This enables healthcare providers to identify high-risk patients, predict potential health issues, and provide targeted interventions to improve patient outcomes and reduce healthcare costs.
Conclusion
In today’s data-driven world, the roles of data analysts and data scientists are crucial in unlocking insights, driving innovation, and shaping the future across diverse industries. While they have distinct responsibilities, the synergy between these roles is essential in providing a comprehensive understanding of data. By working together, data analysts and data scientists can provide valuable insights that inform business strategies and drive growth. As data continues to grow exponentially, the demand for skilled data professionals will only continue to rise, making data analysis and science promising career paths for those who are passionate about uncovering meaningful insights from raw data.