Data science, the field that unlocks the secrets hidden within vast datasets, is undergoing a profound revolution. At its heart lies a powerful ally: Artificial Intelligence (AI). This synergy, a fusion of human ingenuity and machine learning prowess, is redefining the landscape of data analysis, pushing the boundaries of what we can achieve with data. In this article, we will delve into the evolution of AI in data science, exploring its history, role, applications, challenges, and future prospects.
Introduction
Artificial Intelligence (AI) refers to the simulation of human intelligence in machines that are programmed to think and act like humans. It involves creating algorithms that can learn from data, make decisions, and improve over time. Data science, on the other hand, is the process of extracting insights and knowledge from large and complex datasets through various techniques such as statistics, machine learning, and data mining.
The integration of AI in data science has been a game-changer, enabling data scientists to handle massive volumes of data and uncover patterns and insights that were previously impossible to detect. This has led to advancements in various industries, including healthcare, finance, retail, and transportation, to name a few. Let us now take a closer look at the history of AI and its journey towards becoming an integral part of data science.
History of Artificial Intelligence
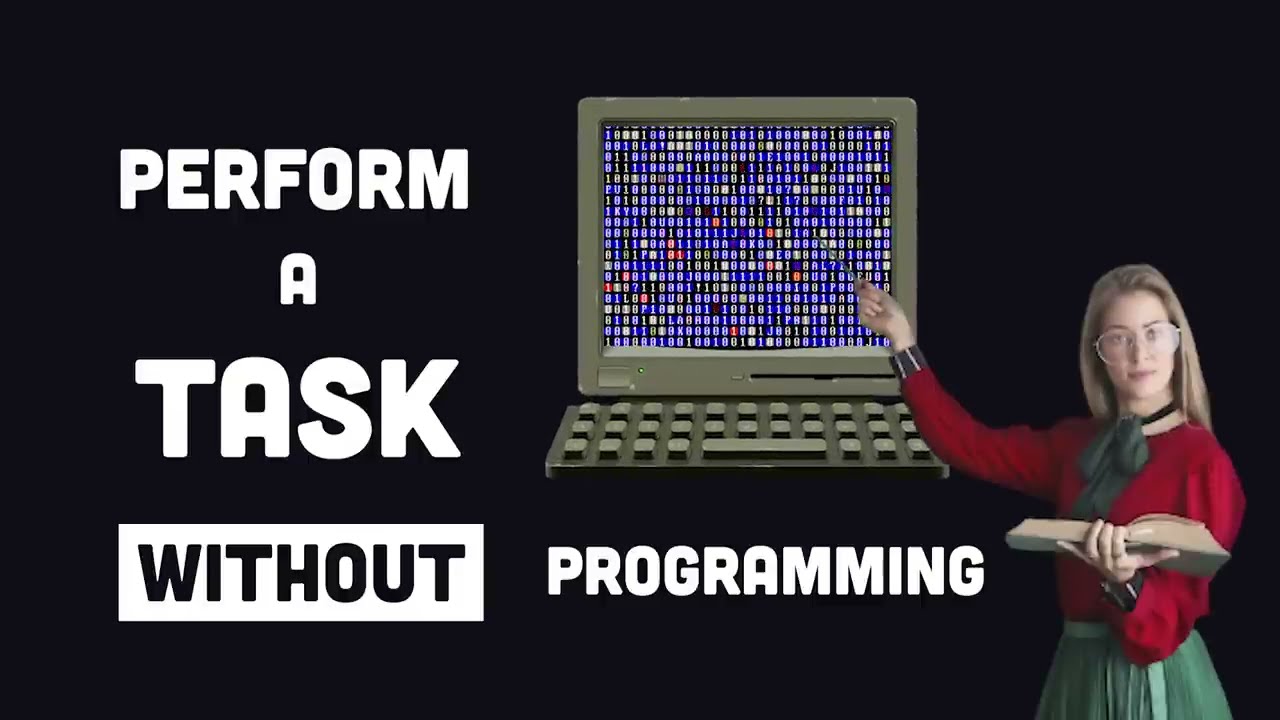
The concept of AI can be traced back to ancient civilizations, where myths and stories depicted artificial beings with human-like intelligence. However, it was not until the 1950s when the term “Artificial Intelligence” was coined by computer scientist John McCarthy, who is considered the father of AI.
During the 1950s and 1960s, AI research was primarily focused on problem-solving and reasoning. This led to the development of symbolic AI, which involved using rules and logic to solve problems. However, this approach had limitations as it could not handle complex and ambiguous problems.
In the 1980s, a new branch of AI called machine learning emerged, which focused on developing algorithms that could learn from data. This marked a significant shift in AI research, with more emphasis on practical applications rather than theoretical concepts. In 1997, IBM’s Deep Blue computer defeated chess grandmaster Garry Kasparov, marking a significant milestone in the advancement of AI.
The early 2000s saw a renewed interest in AI, with the emergence of big data and improved computing power. This led to rapid developments in deep learning, a subfield of machine learning that involves creating artificial neural networks, inspired by the structure and function of the human brain. Today, AI has become an essential part of various technologies such as virtual assistants, self-driving cars, and facial recognition systems, to name a few.
Role of AI in Data Science

AI plays a crucial role in data science, enabling data scientists to handle massive amounts of data and extract meaningful insights. Let us take a look at some of the key roles of AI in data science:
Automating the Mundane
One of the most significant advantages of AI in data science is its ability to automate tedious and repetitive tasks, freeing up data scientists to focus on higher-level analysis. This includes data pre-processing, feature engineering, and model building, to name a few.
Data Pre-processing
Data pre-processing involves cleaning, transforming, and preparing messy datasets for further analysis. This can be a time-consuming and labor-intensive process, involving tasks such as handling missing values, handling outliers, and dealing with inconsistent data formats. With AI, data scientists can automate these tasks, saving hours of manual effort and ensuring a more efficient and accurate data cleaning process.
Feature Engineering
Feature engineering refers to the process of identifying and selecting relevant features from a dataset for model building. This is a crucial step in data science, as the success of a model depends largely on the features used. Traditionally, feature engineering involved extensive trial and error, with data scientists manually selecting and combining features. However, with AI, this process can be automated, with algorithms suggesting optimal feature combinations based on the data.
Model Building
Model building is the heart of data science, where algorithms are designed and trained to make predictions or uncover patterns in data. With AI, data scientists can leverage advanced techniques such as reinforcement learning and deep learning to build sophisticated models that can handle complex data and produce accurate results.
Enhancing Decision-Making
AI has the ability to analyze large and complex datasets at lightning speed, enabling data scientists to make faster and more informed decisions. This is especially beneficial in industries such as finance and healthcare, where time is of the essence, and quick decision-making can have a significant impact. By using AI-powered predictive analytics tools, data scientists can identify trends and patterns that may not be visible to the human eye, helping organizations make strategic decisions and stay ahead of the competition.
Improving Accuracy and Efficiency
Data science involves dealing with huge volumes of data, which can be overwhelming for even the most skilled data scientists. AI algorithms, on the other hand, are not limited by human capacity and can analyze vast amounts of data quickly and accurately. This improves the efficiency of data analysis, ensuring more precise and reliable results.
Applications of AI in Data Science
The integration of AI in data science has led to groundbreaking advancements in various industries. Let us take a look at some of the key applications of AI in data science:
Healthcare
In the field of healthcare, AI has been used for tasks such as disease diagnosis, drug discovery, and patient monitoring. With the help of AI algorithms, medical professionals can analyze patient data and identify patterns and trends that can assist in early detection and prevention of diseases. AI-powered medical devices can also monitor patients in real-time, providing timely alerts in case of any abnormalities.
Finance
In the finance industry, AI has been used for tasks such as fraud detection, risk assessment, and portfolio management. With the help of AI algorithms, financial institutions can sift through vast amounts of data to identify fraudulent activities and improve the accuracy of credit risk assessments. AI-powered chatbots are also being used in banking and insurance to handle customer queries and assist with routine transactions.
Retail
AI has revolutionized the retail industry, with applications such as demand forecasting, personalized marketing, and inventory management. By analyzing customer data, AI algorithms can predict consumer behavior, enabling retailers to optimize their inventory levels and offer personalized recommendations to customers based on their preferences and purchase history.
Transportation
The transportation industry has also seen significant advancements with the integration of AI. Self-driving cars, powered by AI algorithms, have the potential to reduce accidents and improve traffic flow. AI has also been used for route optimization, reducing fuel consumption and improving delivery times in logistics.
Challenges and Ethical Considerations
While AI has proven to be a valuable tool in data science, it also brings along its fair share of challenges and ethical considerations. Let us take a closer look at some of these:
Data Bias
AI algorithms are only as good as the data they are trained on. If the training data is biased, the resulting algorithms will also be biased, leading to inaccurate or discriminatory decisions. This can have serious implications, especially in areas such as healthcare and finance, where AI decisions can significantly impact people’s lives.
Lack of Transparency
Another challenge with AI is the lack of transparency in how algorithms make decisions. Deep learning algorithms, in particular, are often referred to as “black boxes” as it is difficult to understand the reasoning behind their decisions. This makes it challenging for data scientists to explain or justify the results of their models.
Ethical Considerations
The use of AI in data science raises ethical concerns about privacy, security, and the potential misuse of personal data. There have been numerous cases where AI has been used to manipulate public opinion or discriminate against certain groups of people. This calls for ethical guidelines and regulations to ensure responsible and ethical use of AI.
Future of AI in Data Science
The fusion of AI and data science is transforming industries and opening up new possibilities for innovation. As technology continues to advance, we can expect to see even more groundbreaking applications of AI in data science. Some of the key areas that are likely to see significant developments include:
Natural Language Processing (NLP)
NLP involves training algorithms to understand and process human language. With advancements in NLP, we can expect to see more sophisticated virtual assistants and chatbots that can handle complex queries and interactions with humans.
Internet of Things (IoT)
The IoT refers to the network of interconnected devices that collect and exchange data. With the integration of AI, these devices can become even smarter, enabling them to make decisions and take actions without human intervention. This can lead to significant improvements in areas such as smart homes, self-driving cars, and industrial automation.
Explainable AI
As mentioned earlier, one of the challenges with AI is the lack of transparency in its decision-making processes. Explainable AI aims to address this by creating algorithms that can explain how they reached a particular decision, making it easier for data scientists to understand and justify their models.
Conclusion
The evolution of AI in data science has been nothing short of remarkable. It has enabled us to analyze vast amounts of data, make faster and more informed decisions, and unlock insights that were previously hidden. However, as with any powerful tool, AI also brings along its own set of challenges and ethical considerations. It is essential to approach its development and implementation responsibly and ethically to harness its full potential for the betterment of society. As technology continues to advance, we can only imagine what the future holds for this powerful fusion of minds.